les Thèses Soutenues à l'USTO MB
vous cherchez une thèse?
A partir de cette page vous pouvez :
Retourner au premier écran avec les recherches... | Votre compte |
Détail de l'auteur
Auteur MOUHAB Abderrahmane
Documents disponibles écrits par cet auteur



A bayesian decision theory approach to screening and classification , with large samples results / MOUHAB Abderrahmane
Titre : A bayesian decision theory approach to screening and classification , with large samples results Type de document : texte imprimé Auteurs : MOUHAB Abderrahmane, Auteur ; RICHARD A.Johnson, Directeur de thèse Année de publication : 1991 Importance : 111 p. Langues : Anglais (eng) Catégories : Mathématique Mots-clés : Population multivariate normal the predictive denity predictive rule Résumé : Firstly , we address the screening problem where an item is declared to be good if certain of its characteristics y lie within a specified set , often , these criterion variables cannot be measured without damaging the object , the characteristics of an experimental unit are partitioned into criterion variables Y and covariates X useful for screening we develop a bayes screening procedure based on a training set of data which includes the values of both X and Y for a future unit , only X will be observed , our decision theory approach integrates all of the components ‘(1) will be misclassification costs (2) conditional densities of the X and Y given the parameters , and (3) a prior distribution , it establishes that the bayes rule is based on the predictive distribution , we generalize the result to the case of screening into m classes A bivariate normal example illustrates the point that extreme values for the ratio of misclassification costs can produce bayes rules where the objects are always called good whatever the values of the covariates
Secondly we address the classification problem , again using a decision approach we derive an alternative to the classification rule by allowing the parmeters of the underlying distributions to be generated from a prior distribution , the bayes rule is than extended to the m -population classification problem
We derive an asymptotic expansion of the predictive distribution where the coefficients of powers of n -1 remain uniformly bounded in n when they are integrated over the entire sample space , using the result for a first order expansion of the predictive density , we obtain asmtotic bounds for the bayes risk both the classification and the screening problems
A bayesian decision theory approach to screening and classification , with large samples results [texte imprimé] / MOUHAB Abderrahmane, Auteur ; RICHARD A.Johnson, Directeur de thèse . - 1991 . - 111 p.
Langues : Anglais (eng)
Catégories : Mathématique Mots-clés : Population multivariate normal the predictive denity predictive rule Résumé : Firstly , we address the screening problem where an item is declared to be good if certain of its characteristics y lie within a specified set , often , these criterion variables cannot be measured without damaging the object , the characteristics of an experimental unit are partitioned into criterion variables Y and covariates X useful for screening we develop a bayes screening procedure based on a training set of data which includes the values of both X and Y for a future unit , only X will be observed , our decision theory approach integrates all of the components ‘(1) will be misclassification costs (2) conditional densities of the X and Y given the parameters , and (3) a prior distribution , it establishes that the bayes rule is based on the predictive distribution , we generalize the result to the case of screening into m classes A bivariate normal example illustrates the point that extreme values for the ratio of misclassification costs can produce bayes rules where the objects are always called good whatever the values of the covariates
Secondly we address the classification problem , again using a decision approach we derive an alternative to the classification rule by allowing the parmeters of the underlying distributions to be generated from a prior distribution , the bayes rule is than extended to the m -population classification problem
We derive an asymptotic expansion of the predictive distribution where the coefficients of powers of n -1 remain uniformly bounded in n when they are integrated over the entire sample space , using the result for a first order expansion of the predictive density , we obtain asmtotic bounds for the bayes risk both the classification and the screening problems
Exemplaires
Code-barres Cote Support Localisation Section Disponibilité 4838 02-04-130 version papier Bibliothèque USTOMB Thèse de Doctorat Exclu du prêt
BUC USTOMB'Thèses
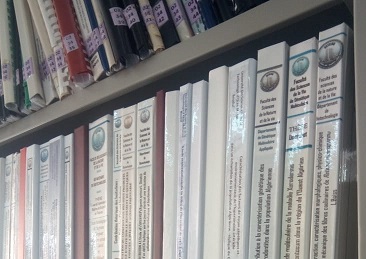
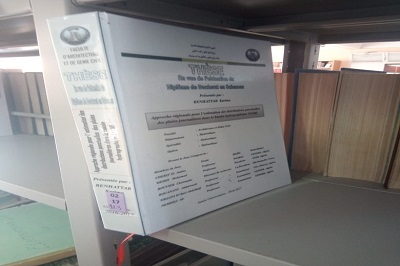
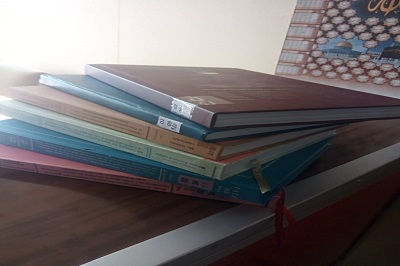
Service Thèse de la BUC met à votre disposition L'ensemble des thèses de doctorat et mémoires de magister soutenues à l'USTO MB
Adresse
BUC USTOMB'ThèsesBibliothèque centrale USTOMB
BP 1505 EL M'Naouer USTO ORAN
Algérie
(213)041627180
contact