les Thèses Soutenues à l'USTO MB
vous cherchez une thèse?
A partir de cette page vous pouvez :
Retourner au premier écran avec les recherches... | Votre compte |
Détail de l'auteur
Auteur CHALOULI Mohammed
Documents disponibles écrits par cet auteur



Development of an Intelligent Health Assessment Application for Bearing Machines / CHALOULI Mohammed
![]()
Titre : Development of an Intelligent Health Assessment Application for Bearing Machines Type de document : texte imprimé Auteurs : CHALOULI Mohammed, Auteur Année de publication : 2022-2023 Accompagnement : CD Langues : Français (fre) Catégories : Electronique:Systèmes intelligents et robotique Mots-clés : Fault feature extraction Failure diagnostic PHM Prognostics and health monitoring Feature fusion Health indicator Trend indicator Bearing Generic prognostics Bearing health assessment
استخراج ميزة الخطأ. تشخيص الفشل التشخيص والرصد الصحي مؤشر الصحة مؤشر الاتجاه تحمل. التكهنات العامة مؤشر الصحةRésumé :
The intense challenge in the industrial sector nowadays imposes manufacturers to optimize the production process to offer competitive quality in short delivery time. These improvements request that the equipment should work in the best conditions as long as possible with near to zero downtime. For that, unplanned breakdown and replacement of good equipment before reaching their tolerable life cycle is a loss, directly influencing the cost of the final product. In such conditions and with the substantial technical evolution of sensors and computing resources in the last decade, new maintenance strategies were developed, allowing the move from corrective to predictive maintenance.
The prognostics & health monitoring (PHM) is in this case the most appropriate maintenance plan. It consists of detecting failures before any damage by monitoring the equipment's health and anticipating failure. In addition, prior knowledge offers the possibility of purchasing spare parts, planning maintenance tasks, avoiding unplanned breakdowns, and optimizing equipment durability and maintenance activities.
This research focuses on the rotating machines' prognostic, especially the bearing component. The proposed approach is based on extracting appropriate multiple domain features from the raw signals (mainly those related to vibration). Then, these features are reduced using a cross-correlation filter before being projected onto two dimensions space using a Self-Organizing Map (SOM). The SOM is compared with multiple values extracted from the Principal Component Analysis (PCA) to select the optimal ones by using some reduction techniques. Hereafter, the result is considered the Health Indicator (HI), used for fault detection. The fault detection allows for the Remaining Useful Lifetime (RUL) estimation where the fault represents the beginning of the RUL, and the Failure means its end. Thus, to predict the failure time for a new component, a Trend Indicator (TI) is built from the residual of the Empirical Model Decomposition (EMD) to get a monotonic signal. Finally, the Gaussian Process Regression (GPR) is used in this case study as a regression of the TI, where it provides a high-accuracy prediction of the RUL. Since the quality of the RUL estimation relies directly on the selected features, this work focuses mainly on relevant feature extraction and selection. The proposed method is tested on various real benchmarked bearing datasets to validate our work.
يفرض التحدي الشديد في القطاع الصناعي في الوقت الحاضر على الشركة المصنعة تحسين عملية الإنتاج من أجل توفير الجودة خلال وقت تسليم تنافسي. يتطلب هذا التحسين أن تعمل المعدات في أفضل الظروف لأطول فترة ممكنة دون توقف. لذلك، فإن التوقف غير المخطط له وكذلك استبدال المعدات الجيدة قبل الوصول إلى دورة حياتها المقبولة يمثلان خسارة كبيرة، مما يؤثر بشكل مباشر على تكلفة المنتج النهائي. في مثل هذه الحالة ومع التطور التقني الهائل لأجهزة الاستشعار والمعدات في العقد الماضي، تم تطوير استراتيجيات صيانة جديدة تسمح بالانتقال من الصيانة التصحيحية إلى الصيانة التنبؤية.
تعد خطة التوقعات والرصد الصحي (PHM) أنسب خطة صيانة في هذه الحالة، والتي تتكون من اكتشاف الأعطاب قبل حدوث أي ضرر، من خلال مراقبة الحالات الصحية للمعدات وتوقع تعطلها. وبالإضافة إلى ذلك، ، توفر المعرفة المسبقة إمكانية شراء قطع الغيار في الوقت المناسب، فضلا عن تخطيط مهام الصيانة، وتجنب التعطل غير المخطط له.
في هذا البحث، نركز على تشخيص الآلات الدوارة وخاصة على عنصر المحمل. يعتمد النهج المقترح على استخراج الخصائص في مجالات متعددة من الاهتزازات. بعد ذلك، يتم تقليل الخصائص باستخدام مرشح الارتباط المتقاطع قبل إسقاطها في مساحة ثنائية الأبعاد باستخدام خريطة ذاتية التنظيم (SOM). لاختيار تقنية تخفيض الخصائص المناسبة، تتم مقارنة SOM بالقيم المتعددة المستخرجة من تحليل المكون الرئيسي (PCA). تعتبر النتيجة المؤشر الصحي (HI)، والذي سيتم استخدامه لاكتشاف العطب. ويمكن الكشف عن العمر النافع المتبقي (RUL)، الذي يتمثل في الوقت المستغرق من بداية العطب وينتهي بعطل الآلة. وبالتالي، ومن أجل التنبؤ بوقت عطب المكون الجديد، يتم إنشاء مؤشر الاتجاه (TI) من بقايا تحليل النموذج التجريبي (EMD) من أجل الحصول على إشارة رتيبة. أخيرًا، يتم استخدام تراجع عملية Gauss في هذه الدراسة كتراجع لمؤشر الاتجاه حيث توفر تنبؤًا دقيقًا للغاية لـ RUL. نظرًا لأن جودة تقدير RUL تعتمد بشكل مباشر على الخصائص المختارة، فإن هذا العمل يركز بشكل أساسي على استخراج الخصائص التي لها تأثير كبير في تحديد الحالة الصحية للآلة. من أجل التحقق من صحة عملنا، يتم اختبار الطريقة المقترحة على عدة مجموعات من بيانات المحمل المرجعية.
Directeur de thèse : BERRACHED Nasreddine Development of an Intelligent Health Assessment Application for Bearing Machines [texte imprimé] / CHALOULI Mohammed, Auteur . - 2022-2023 . - + CD.
Langues : Français (fre)
Catégories : Electronique:Systèmes intelligents et robotique Mots-clés : Fault feature extraction Failure diagnostic PHM Prognostics and health monitoring Feature fusion Health indicator Trend indicator Bearing Generic prognostics Bearing health assessment
استخراج ميزة الخطأ. تشخيص الفشل التشخيص والرصد الصحي مؤشر الصحة مؤشر الاتجاه تحمل. التكهنات العامة مؤشر الصحةRésumé :
The intense challenge in the industrial sector nowadays imposes manufacturers to optimize the production process to offer competitive quality in short delivery time. These improvements request that the equipment should work in the best conditions as long as possible with near to zero downtime. For that, unplanned breakdown and replacement of good equipment before reaching their tolerable life cycle is a loss, directly influencing the cost of the final product. In such conditions and with the substantial technical evolution of sensors and computing resources in the last decade, new maintenance strategies were developed, allowing the move from corrective to predictive maintenance.
The prognostics & health monitoring (PHM) is in this case the most appropriate maintenance plan. It consists of detecting failures before any damage by monitoring the equipment's health and anticipating failure. In addition, prior knowledge offers the possibility of purchasing spare parts, planning maintenance tasks, avoiding unplanned breakdowns, and optimizing equipment durability and maintenance activities.
This research focuses on the rotating machines' prognostic, especially the bearing component. The proposed approach is based on extracting appropriate multiple domain features from the raw signals (mainly those related to vibration). Then, these features are reduced using a cross-correlation filter before being projected onto two dimensions space using a Self-Organizing Map (SOM). The SOM is compared with multiple values extracted from the Principal Component Analysis (PCA) to select the optimal ones by using some reduction techniques. Hereafter, the result is considered the Health Indicator (HI), used for fault detection. The fault detection allows for the Remaining Useful Lifetime (RUL) estimation where the fault represents the beginning of the RUL, and the Failure means its end. Thus, to predict the failure time for a new component, a Trend Indicator (TI) is built from the residual of the Empirical Model Decomposition (EMD) to get a monotonic signal. Finally, the Gaussian Process Regression (GPR) is used in this case study as a regression of the TI, where it provides a high-accuracy prediction of the RUL. Since the quality of the RUL estimation relies directly on the selected features, this work focuses mainly on relevant feature extraction and selection. The proposed method is tested on various real benchmarked bearing datasets to validate our work.
يفرض التحدي الشديد في القطاع الصناعي في الوقت الحاضر على الشركة المصنعة تحسين عملية الإنتاج من أجل توفير الجودة خلال وقت تسليم تنافسي. يتطلب هذا التحسين أن تعمل المعدات في أفضل الظروف لأطول فترة ممكنة دون توقف. لذلك، فإن التوقف غير المخطط له وكذلك استبدال المعدات الجيدة قبل الوصول إلى دورة حياتها المقبولة يمثلان خسارة كبيرة، مما يؤثر بشكل مباشر على تكلفة المنتج النهائي. في مثل هذه الحالة ومع التطور التقني الهائل لأجهزة الاستشعار والمعدات في العقد الماضي، تم تطوير استراتيجيات صيانة جديدة تسمح بالانتقال من الصيانة التصحيحية إلى الصيانة التنبؤية.
تعد خطة التوقعات والرصد الصحي (PHM) أنسب خطة صيانة في هذه الحالة، والتي تتكون من اكتشاف الأعطاب قبل حدوث أي ضرر، من خلال مراقبة الحالات الصحية للمعدات وتوقع تعطلها. وبالإضافة إلى ذلك، ، توفر المعرفة المسبقة إمكانية شراء قطع الغيار في الوقت المناسب، فضلا عن تخطيط مهام الصيانة، وتجنب التعطل غير المخطط له.
في هذا البحث، نركز على تشخيص الآلات الدوارة وخاصة على عنصر المحمل. يعتمد النهج المقترح على استخراج الخصائص في مجالات متعددة من الاهتزازات. بعد ذلك، يتم تقليل الخصائص باستخدام مرشح الارتباط المتقاطع قبل إسقاطها في مساحة ثنائية الأبعاد باستخدام خريطة ذاتية التنظيم (SOM). لاختيار تقنية تخفيض الخصائص المناسبة، تتم مقارنة SOM بالقيم المتعددة المستخرجة من تحليل المكون الرئيسي (PCA). تعتبر النتيجة المؤشر الصحي (HI)، والذي سيتم استخدامه لاكتشاف العطب. ويمكن الكشف عن العمر النافع المتبقي (RUL)، الذي يتمثل في الوقت المستغرق من بداية العطب وينتهي بعطل الآلة. وبالتالي، ومن أجل التنبؤ بوقت عطب المكون الجديد، يتم إنشاء مؤشر الاتجاه (TI) من بقايا تحليل النموذج التجريبي (EMD) من أجل الحصول على إشارة رتيبة. أخيرًا، يتم استخدام تراجع عملية Gauss في هذه الدراسة كتراجع لمؤشر الاتجاه حيث توفر تنبؤًا دقيقًا للغاية لـ RUL. نظرًا لأن جودة تقدير RUL تعتمد بشكل مباشر على الخصائص المختارة، فإن هذا العمل يركز بشكل أساسي على استخراج الخصائص التي لها تأثير كبير في تحديد الحالة الصحية للآلة. من أجل التحقق من صحة عملنا، يتم اختبار الطريقة المقترحة على عدة مجموعات من بيانات المحمل المرجعية.
Directeur de thèse : BERRACHED Nasreddine Exemplaires
Code-barres Cote Support Localisation Section Disponibilité 9046 02-09-633 Version numérique et papier Bibliothèque USTOMB Thèse de Doctorat Exclu du prêt Documents numériques
02-09-633.pdfAdobe Acrobat PDF
BUC USTOMB'Thèses
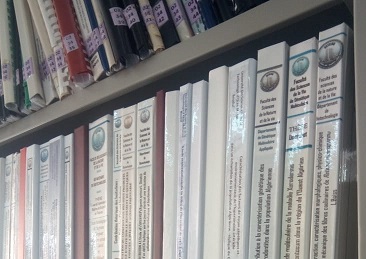
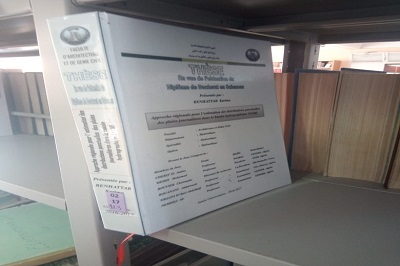
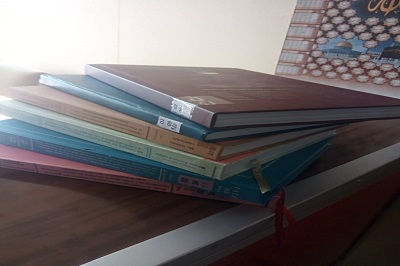
Service Thèse de la BUC met à votre disposition L'ensemble des thèses de doctorat et mémoires de magister soutenues à l'USTO MB
Adresse
BUC USTOMB'ThèsesBibliothèque centrale USTOMB
BP 1505 EL M'Naouer USTO ORAN
Algérie
(213)041627180
contact